Search
information technology and software
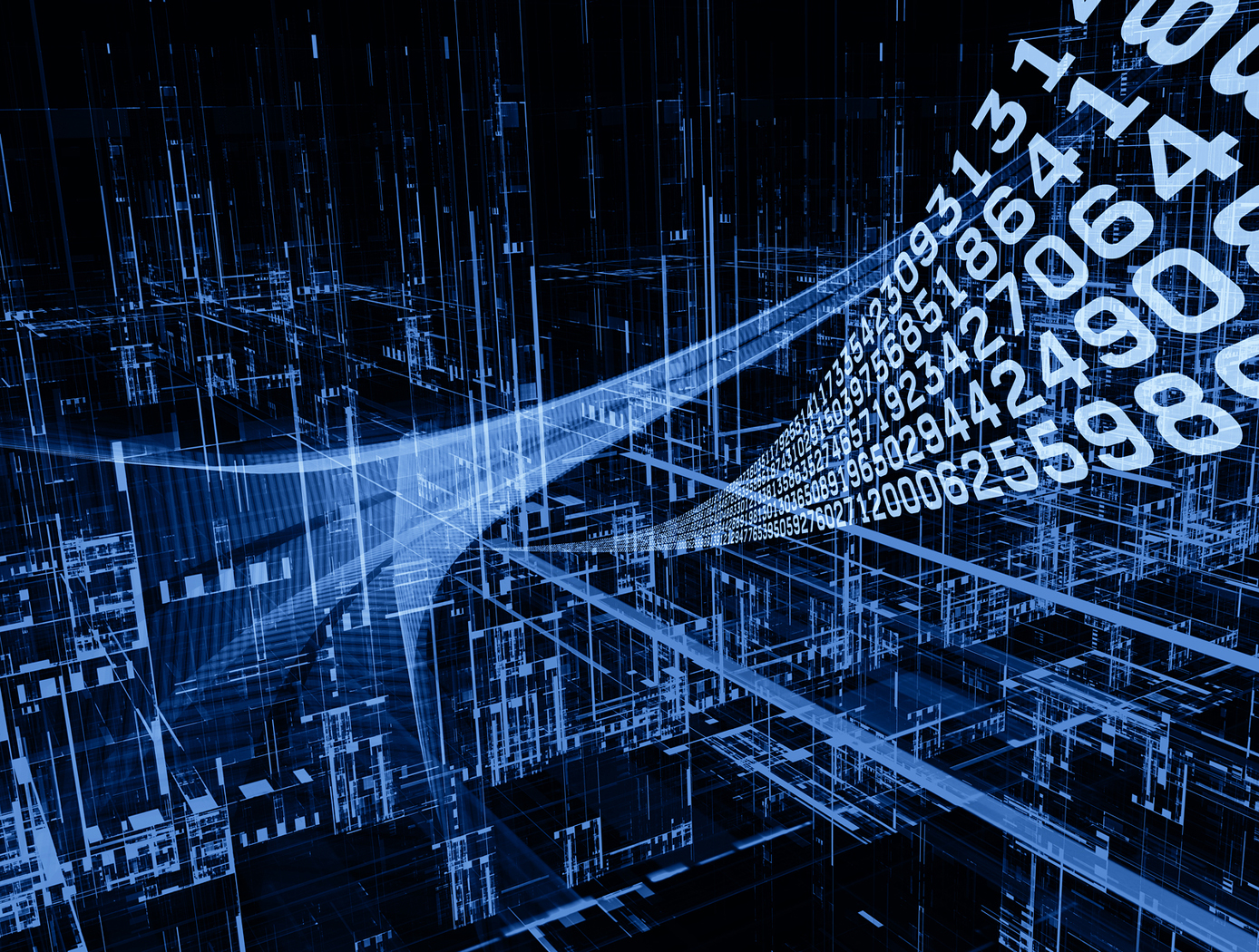
Enhancing Fault Isolation and Detection for Electric Powertrains of UAVs
The tool developed through this work merges information from the electric propulsion system design phase with diagnostic tools. Information from the failure mode and effect analysis (FMEA) from the system design phase is embedded within a Bayesian network (BN). Each node in the network can represent either a fault, failure mode, root cause or effect, and the causal relationships between different elements are described through the connecting edges.
This novel approach can help Fault Detection and Isolation (FDI), producing a framework capable of isolating the cause of sub-system level fault and degradation.
This system:
Identifies and quantifies the effects of the identified hazards, the severity and probability of their effects, their root cause, and the likelihood of each cause;
Uses a Bayesian framework for fault detection and isolation (FDI);
Based on the FDI output, estimates health of the faulty component and predicts the remaining useful life (RUL) by also performing uncertainty quantification (UQ);
Identifies potential electric powertrain hazards and performs a functional hazard analysis (FHA) for unmanned aerial vehicles (UAVs)/Urban Air Mobility (UAM) vehicles.
Despite being developed for and demonstrated with an application to an electric UAV, the methodology is generalized and can be implemented in other domains, ranging from manufacturing facilities to various autonomous vehicles.
robotics automation and control
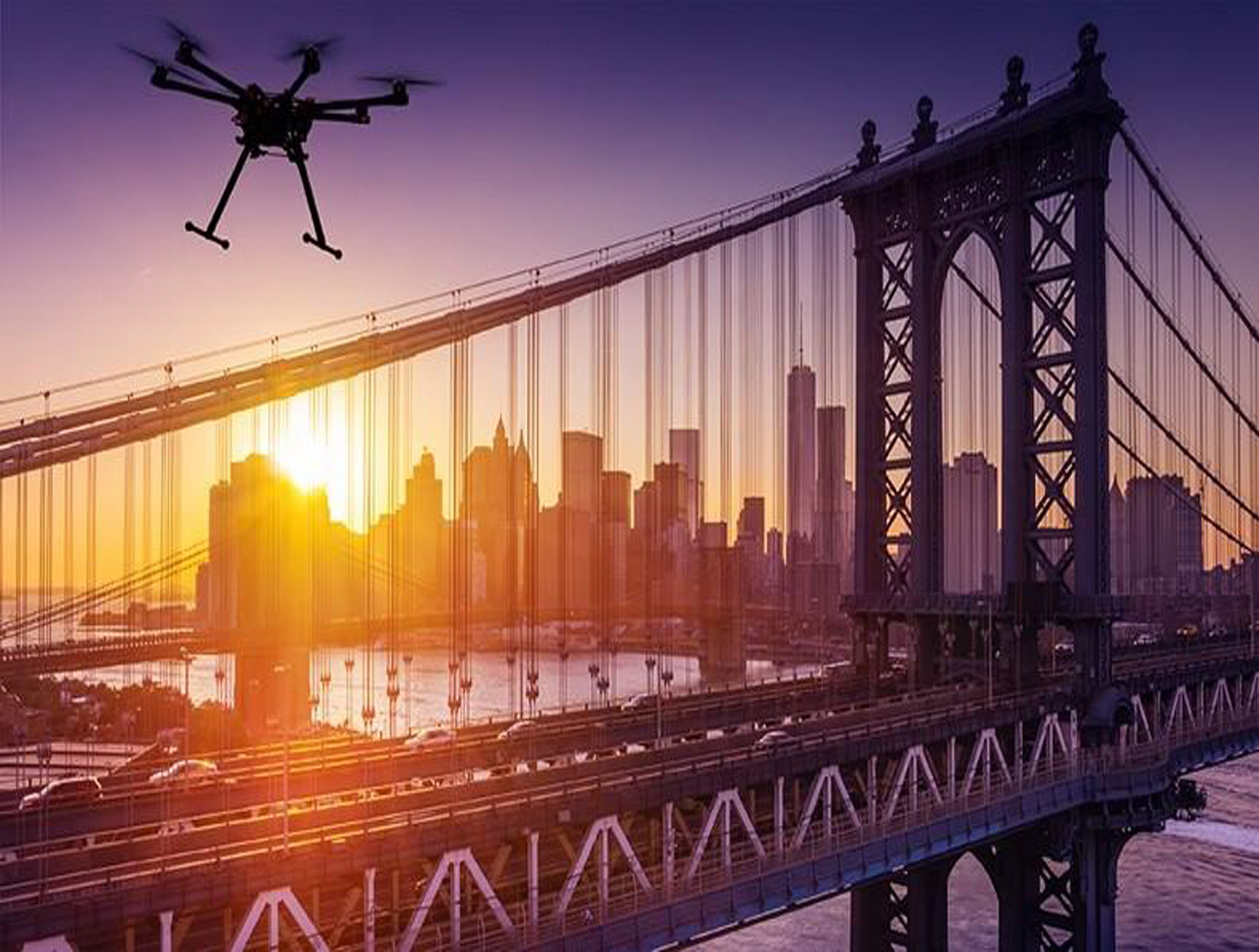
Airborne Machine Learning Estimates for Local Winds and Kinematics
The MAchine learning ESTimations for uRban Operations (MAESTRO) system is a novel approach that couples commodity sensors with advanced algorithms to provide real-time onboard local wind and kinematics estimations to a vehicle's guidance and navigation system. Sensors and computations are integrated in a novel way to predict local winds and promote safe operations in dynamic urban regions where Global Positioning System/Global Navigation Satellite System (GPS/GNSS) and other network communications may be unavailable or are difficult to obtain when surrounded by tall buildings due to multi-path reflections and signal diffusion. The system can be implemented onboard an Unmanned Aerial Systems (UAS) and once airborne, the system does not require communication with an external data source or the GPS/GNSS. Estimations of the local winds (speed and direction) are created using inputs from onboard sensors that scan the local building environment. This information can then be used by the onboard guidance and navigation system to determine safe and energy-efficient trajectories for operations in urban and suburban settings. The technology is robust to dynamic environments, input noise, missing data, and other uncertainties, and has been demonstrated successfully in lab experiments and computer simulations.
Information Technology and Software
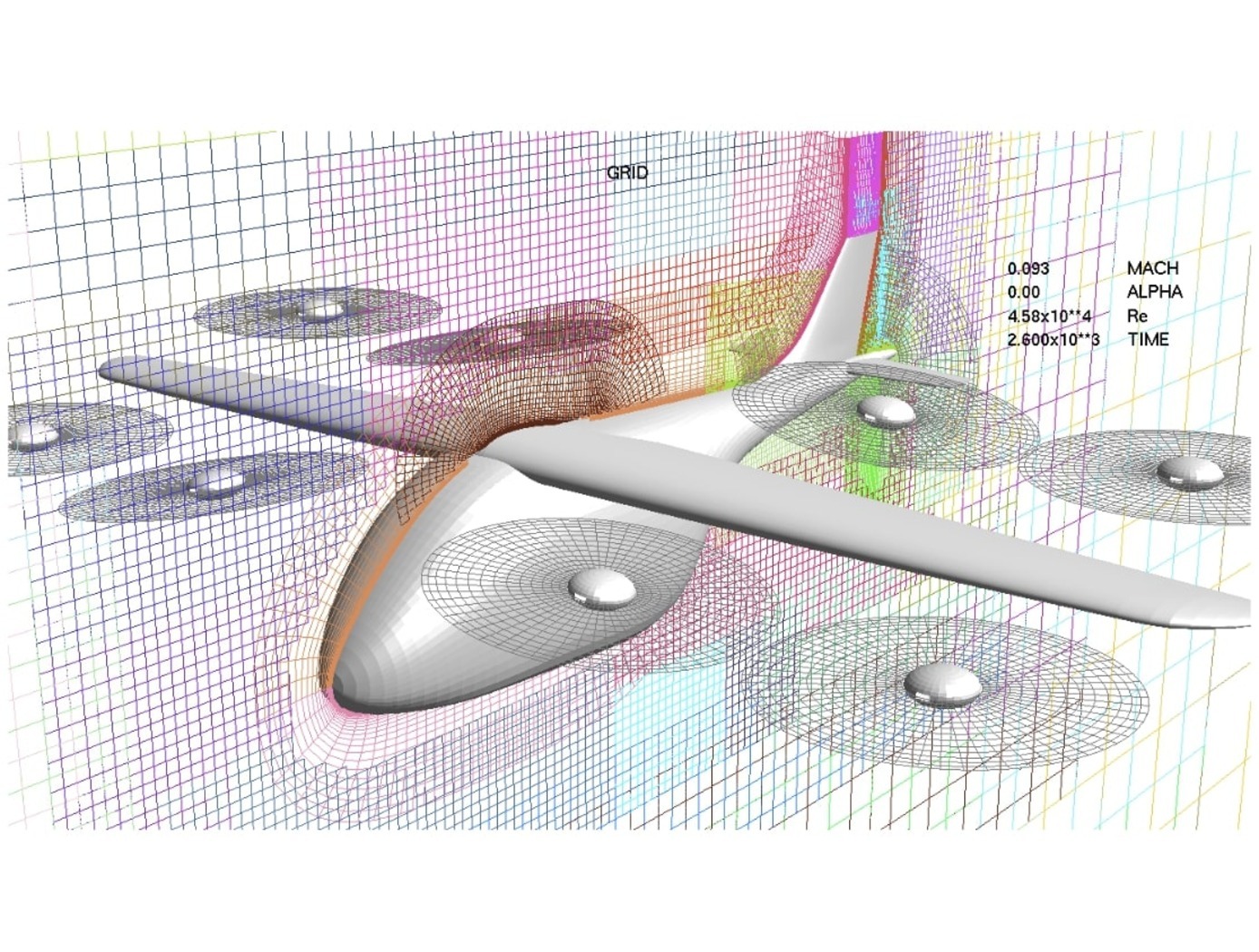
Rapid Aero Modeling for Computational Experiments
RAM-C interfaces with computational software to provide test logic and manage a unique process that implements three main bodies of theory: (a) aircraft system identification (SID), (b) design of experiment (DOE), and (c) CFD. SID defines any number of alternative estimation methods that can be used effectively under the RAM-C process (e.g., machine learning techniques, regression, neural nets, fuzzy modeling, etc.). DOE provides a statistically rigorous, sequential approach that defines the test points required for a given model complexity. Typical DOE test points are optimized to reduce either estimation error or prediction error. CFD provides a large range of fidelity for estimating aircraft aerodynamic responses. In initial implementations, NASA researchers “wrapped” RAM-C around OVERFLOW, a NASA-developed high-fidelity CFD flow solver. Alternative computational software requiring less time and computational resources could be also utilized.
RAM-C generates reduced-order aerodynamic models of aircraft. The software process begins with the user entering a desired level of fidelity and a test configuration defined in terms appropriate for the computational code in use. One can think of the computational code (e.g., high-fidelity CFD flow solver) as the “test facility” with which RAM-C communicates with to guide the modeling process. RAM-C logic determines where data needs to be collected, when the mathematical model structure needs to increase in order, and when the models satisfy the desired level of fidelity.
RAM-C is an efficient, statistically rigorous, automated testing process that only collects data required to identify models that achieve user-defined levels of fidelity – streamlining the modeling process and saving computational resources and time. At NASA, the same Rapid Aero Modeling (RAM) concept has also been applied to other “test facilities” (e.g., wind tunnel test facilities in lieu of CFD software).
Aerospace
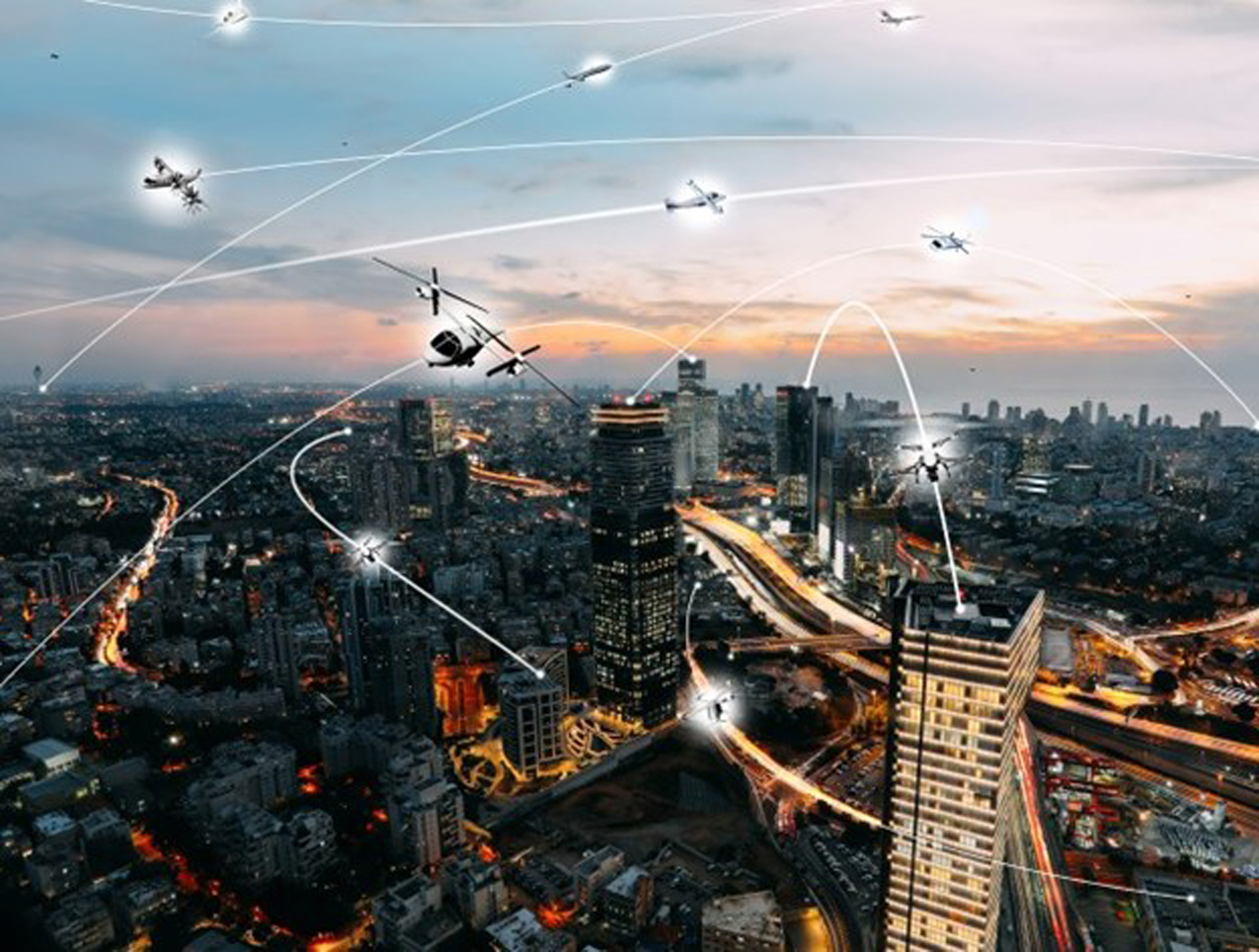
Vision-based Approach and Landing System (VALS)
The novel Vision-based Approach and Landing System (VALS) provides Advanced Air Mobility (AAM) aircraft with an Alternative Position, Navigation, and Timing (APNT) solution for approach and landing without relying on GPS. VALS operates on multiple images obtained by the aircraft’s video camera as the aircraft performs its descent. In this system, a feature detection technique such as Hough circles and Harris corner detection is used to detect which portions of the image may have landmark features. These image areas are compared with a stored list of known landmarks to determine which features correspond to the known landmarks. The world coordinates of the best matched image landmarks are inputted into a Coplanar Pose from Orthography and Scaling with Iterations (COPOSIT) module to estimate the camera position relative to the landmark points, which yields an estimate of the position and orientation of the aircraft. The estimated aircraft position and orientation are fed into an extended Kalman filter to further refine the estimation of aircraft position, velocity, and orientation. Thus, the aircraft’s position, velocity, and orientation are determined without the use of GPS data or signals. Future work includes feeding the vision-based navigation data into the aircraft’s flight control system to facilitate aircraft landing.
Aerospace
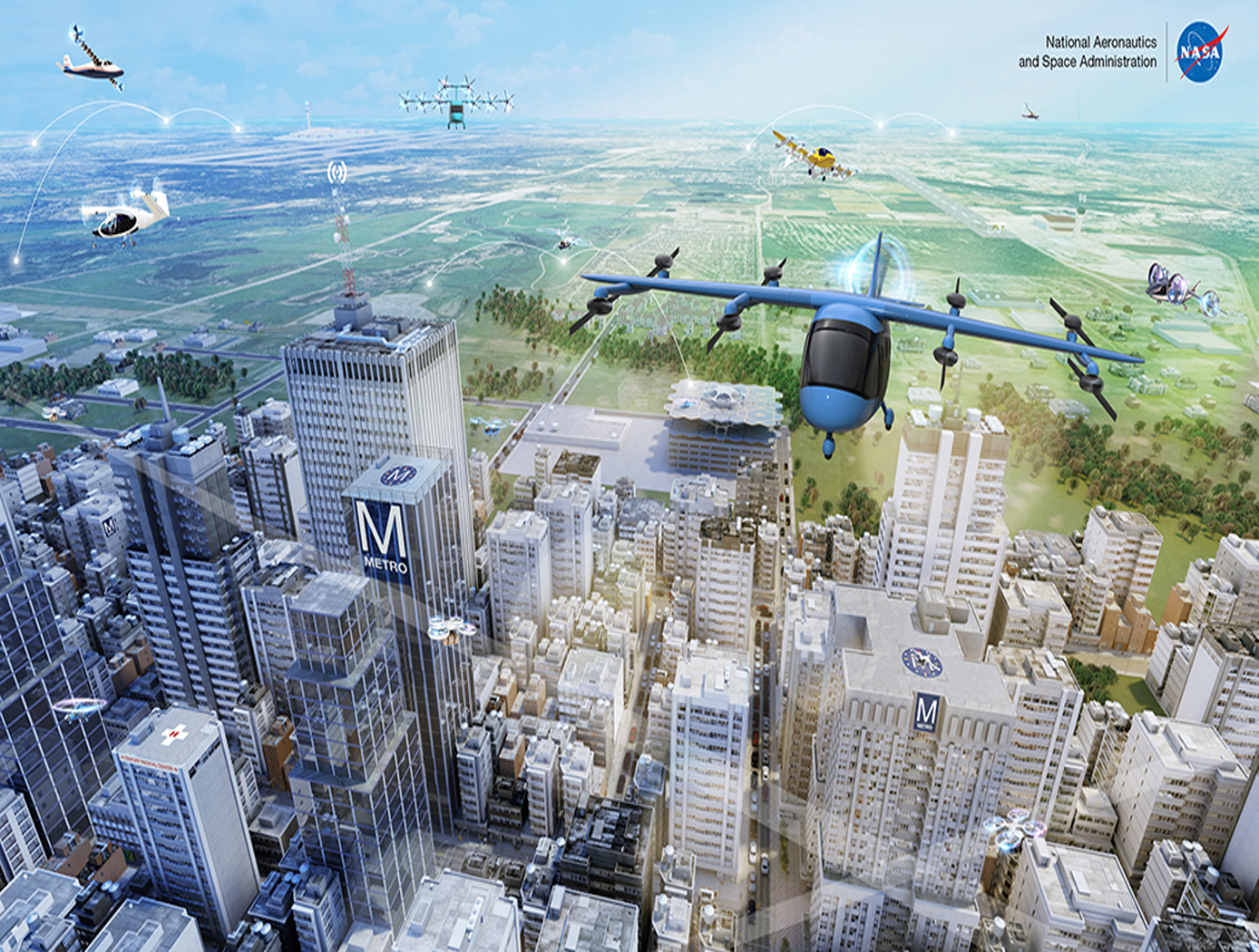
Wind-Optimal Cruise Airspeed Mode for Flight Management Systems (FMS)
The novel approach for optimizing airspeed for both actual and predicted wind conditions in electric Vertical Takeoff and Landing (eVTOL) aircraft with Distributed Electric Propulsion (DEP) systems includes the process of creating a lookup table for wind‐optimal airspeed as a function of wind magnitude, considering the direction of the wind relative to the cruise segment, considering the cruise altitude for an aircraft type, and incorporating the wind-optimal airspeed lookup table in the performance database for real‐time access by the Flight Management Systems (FMS) to predict wind-optimal airspeed at waypoints of the flight plan. The target wind‐optimal airspeed is updated in real-time throughout the cruise portion of a flight.
In a test of the wind-optimal airspeed targeting technique using a multi-rotor aircraft model, results obtained show benefits of flying at the wind‐optimal cruise airspeed compared to the best‐range airspeed. In headwind conditions, energy consumption was reduced by up to 7.5%, and flight duration was reduced by up to 28%. Under uncertain wind magnitudes, flying at wind-optimal airspeed offered lower variability and higher predictability in energy consumption than flying at best‐range airspeed.